
In the changing world of machine learning there’s a term that’s been creating a buzz “GAN” or Generative Adversarial Network. These clever algorithms use networks in a way allowing them to learn and create without human guidance. In this article we’ll delve deeper into the realm of GANs exploring how they function and their wide range of applications. From generating looking faces to transforming images from day, to night GANs have truly made an impact.
The Genesis of GANs
The abbreviation GAN stands for Generative Adversarial Network, which perfectly captures the essence of these machine learning algorithms. GANs are considered ‘generative’ because once they are trained on a dataset they possess an ability to produce examples that closely resemble what they’ve learned. Imagine training a GAN using a dataset containing millions of face photos; it can then generate new faces that appear as if they were taken by a camera.
What sets GANs apart, from machine learning algorithms is their ability to learn without supervision. Unlike ML algorithms that require guidance and supervision to understand data and determine if their answers are correct GANs are unique because they can teach themselves.
This process of self learning occurs through the interaction of two networks that compete against each other which is why its called ‘adversarial.’
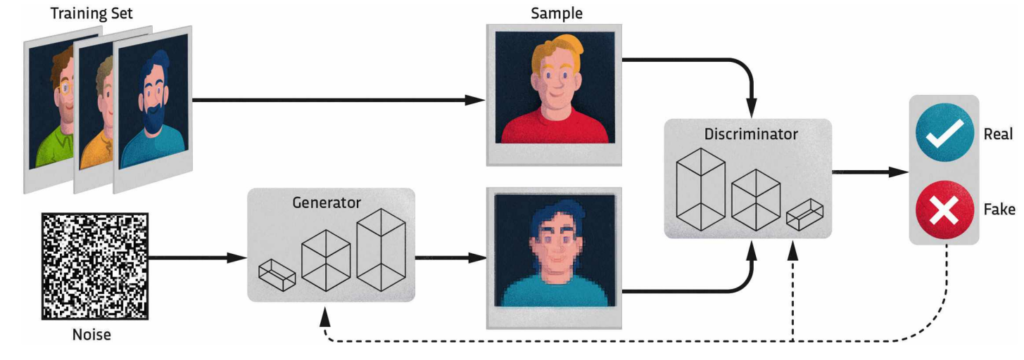
The Duel of Neural Networks
Within this setup the two neural networks in a GAN have roles. The first network, known as the generator learns by studying the training data and aims to create something such as images or other types of data. On the hand the second network acts as the discriminator whose task’s to differentiate between what the generator produces and the original training dataset. The beauty of this arrangement lies in how the generator network receives a reward every time it successfully deceives or fools the discriminator while on the hand the discriminator is rewarded for not being fooled. Through this process both networks continuously improve with their rewards maximized.
The Result: Mastery in Generation
It is this competition between the generator and discriminator that makes GANs truly remarkable. With time the first network becomes exceptionally skilled at generating outputs that’re incredibly similar to the training data. The GAN achieves an ability to replicate and create data that captures and retains key characteristics and essence from its source material.
Beyond the Basics: Enhancing GANs
What amplifies excitement, about GANs further is their adaptability.
When you provide information to a Generative Adversarial Network (GAN) or introduce variations, in its dataset the GAN can accomplish feats. For instance if you give a GAN context like “this image’s a painting by Pollock” or “this photo was taken at night ” it has the ability to generate artwork or transform images with precision.
A Versatile Tool: Applications of GANs
The applications of GANs are diverse. Hold promise. Here are a few examples showcasing how GANs are making advancements in domains;
- Image Enhancement: GANs can take low resolution images and produce higher resolution versions that exhibit remarkable clarity. This technology is particularly valuable in fields such as imaging, where improving the quality of images is crucial.
- Artistic Transformations: GANs have been successfully employed in generating artwork. They can take a sketch. Convert it into a detailed and visually captivating painting even imitating the styles of renowned artists like Van Gogh or Picasso.
- Image to Image Conversion: One area where GANs excel is transforming images from one style to another. For example they can change a scene into a nighttime setting or convert summer landscapes into enchanting winter wonderlands. Such transformations have implications across industries such, as entertainment, design and architecture.
- Data Augmentation: In fields, like machine learning and data science GANs have the ability to generate data points expanding datasets for more thorough and robust training purposes.
- Face Generation: GANs are widely used for creating faces, applicable in various areas such as computer graphics, gaming and even biometric security.
The Road Ahead
The world of GANs is constantly. As they continue to progress we can expect more incredible applications. From transforming the process to enhancing the capabilities of machine learning models GANs are a tool with immense potential.
As researchers and innovators uncover the secrets of these networks further we can anticipate a future where GANs play an increasingly significant role in our everyday lives. The ability to precisely create, transform and generate data opens up a world of possibilities—a journey to witness.
Conclusion
Generative Adversarial Networks (GANs) mark a milestone in the realm of machine learning. Their self learning nature combined with their approach allows them to generate data that closely resembles what they have learned from training datasets. With applications ranging from image enhancement to transformations GANs prove themselves as tools, with a wide range of uses. As we gaze into the future GANs hold the potential to reshape the realm of machine learning and unleash creativity. The remarkable precision with which they can create data and transform existing content presents thrilling prospects across a multitude of fields. We are just scratching the surface of their possibilities as the saga of GANs unfolds with innovation, adaptability and limitless horizons.